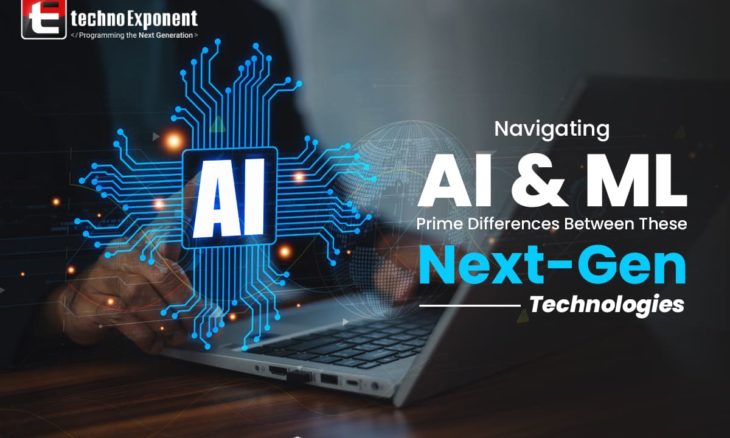
AI and ML are both buzzing topics in the 21st century’s technological landscape that we often use interchangeably. But the reality is both these are distinct topics sharing a profound connection with each other, In simple words, AI ( Artificial Intelligence) denotes an umbrella term for the techniques and strategies that we use to make machines act like humans. Whereas, ML ( Machine Learning) is the science of algorithm development and statistical models that computers use to do such complex tasks without explicit instructions. Dig deeper into the blog to get a detailed notion about their difference and their usage.
Defining AI
Artificial Intelligence, often called AI, is an exciting field focused on creating computers and robots that can imitate and even surpass human abilities. These AI-powered systems can analyze data, provide information, and take actions without needing humans to intervene.
In today’s world, AI plays a central role in many technologies we use daily, like smart devices and voice assistants like Apple’s Siri.
Companies use AI techniques like understanding human language (natural language processing) and interpreting images (computer vision), to make our lives more convenient and efficient.
Defining ML
Machine Learning serves as a crucial path toward achieving Artificial Intelligence. Within the realm of AI, Machine Learning employs algorithms to autonomously uncover insights and identify patterns in data, continually refining its decision-making capabilities.
By delving into Machine Learning, developers can overcome the boundaries of enhancing a computer system’s perception, cognition, and decision-making prowess.
One step further, deep learning, an advanced facet of Machine Learning uses expansive neural networks that mimic human brain function. These networks excel at dissecting intricate patterns and making predictions without requiring human intervention.
Diversified Usage of AI & ML in Different Sectors
AI and ML have found diverse and valuable applications across various sectors, revolutionizing outcomes.
In the banking sector, AI and ML are used for fraud detection and prevention. These technologies can analyze vast datasets to identify irregularities and potential threats in real-time, safeguarding financial transactions and customer assets.
Healthcare benefits from AI and ML through enhanced diagnostics. These technologies assist doctors in interpreting medical images, predicting disease outcomes, and personalizing treatment plans, ultimately improving patient care and reducing errors.
AI and ML have made a profound mark on the business landscape, reducing expenses through automation and extracting valuable insights from vast datasets. Consequently, an increasing number of companies are integrating AI into their operations.
In a 2020 study by NewVantage Partners, 91.5% of surveyed firms indicated continuous AI advancements recognizing its transformative power.
Prime Difference Between AI & ML
Goals
AI makes machines enabled to perform complex human tasks efficiently. These tasks cover several activities, starting from learning and problem-solving to pattern recognition. AI strives to replicate human-like intelligence in machines, making them adaptable and capable of handling various tasks.
The primary goal of ML is to analyze large datasets and identify patterns using statistical models. ML focuses on making predictions or decisions based on data, often providing a confidence level or probability of correctness for its results.
Requirements
ML needs an extensive dataset consisting of several hundred data points for training. Also, they need sufficient computational power to run efficiently. Depending on the specific application, a single server instance or a small cluster can suffice.
In contrast, the infrastructure requirements for AI can vary significantly depending on the complexity of the task. High-computing use cases may demand thousands of machines working together to achieve intricate goals. Prebuilt AI functions are available in this context as well, providing accessibility and scalability to businesses with varying resource needs.
Learning Capability
AI systems focus on replicating human-like intelligence, including reasoning, understanding context, and adapting to new situations. They may employ various techniques beyond just learning from data, such as rule-based reasoning and expert systems.
ML systems primarily learn from data. They become better at tasks as they are exposed to more data, improving their ability to make predictions or decisions within the specific domain they were trained for.
Human Intervention
AI systems don’t need human interference necessarily for performing. Some AI systems, like virtual assistants, may interact with humans in real-time, while others, like autonomous vehicles, operate autonomously.
In contrast, ML systems typically require human intervention for training and data preparation. While they can make predictions autonomously once trained, they rely on humans for initial setup and continuous maintenance.
Implementations
Creating an ML solution involves selecting and preparing a training dataset. Data scientists choose a suitable ML strategy or model, such as linear regression or decision trees, and feed the model with relevant data features. Continuous refinement of the dataset with updated information and error checking is essential to improve model accuracy.
In contrast, developing AI products can be more intricate, requiring extensive research and development efforts. Prebuilt AI solutions are there that have gone through deep research are now available for integration via APIs into various products and services. These prebuilt AI functions simplify the development process and enable businesses to leverage AI capabilities without the required expertise.
Conclusion
In conclusion, the dynamic duo of Artificial Intelligence and Machine Learning has undeniably reshaped the technological landscape of today. Their reciprocal relationship, with AI’s boundless potential and ML’s data-driven precision, has already left an indelible mark on several industries ranging from healthcare to finance. Looking forward, these interconnected technologies promise not just incremental but groundbreaking advancements, ensuring that the future will be marked by innovations going beyond our imagination.