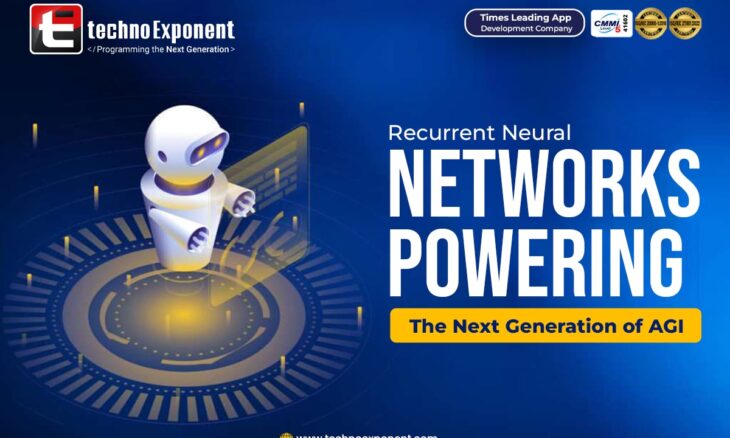
Artificial Intelligence (AI) is evolving, but there’s one particular type of AI model pushing boundaries and bringing us closer to what we imagine as true artificial general intelligence (AGI): the Recurrent Neural Network, or RNN. To understand the power of RNNs, let’s explore what they are, why they’re unique, and how they’re fueling advancements in AI and AGI—making technology smarter, more flexible, and capable of learning and adapting on its own.
What Makes RNNs Different?
Most AI models handle data in a way that’s efficient for certain tasks but lacks context over time. For example, if you trained an AI to identify objects in images, it could tell you if there’s a cat in one picture and a car in the next, but it wouldn’t remember the car in a way that informs how it interprets the next image. These types of models treat each piece of data independently, which works well for some tasks but doesn’t mimic how humans think.
RNNs are different. They process data sequentially—one step after another—which means they remember information over time and can make connections between what they’ve seen before and what they’re seeing now. This ability to retain context and “remember” things is what makes RNNs especially powerful, particularly for tasks where understanding a sequence is crucial, like language, video processing, or predicting trends.
How RNNs Are Shaping AI
Recurrent Neural Networks are foundational for applications that require AI to process and understand sequences. Think about activities like translating languages, driving a car, or recommending a movie. Each of these requires the AI to consider what has happened before to make accurate predictions or suggestions.
Some key abilities make RNNs especially impactful:
1. Sequential Learning – Remembering Context, Just Like Humans
One of the defining characteristics of RNNs is their ability to process information in a specific order. This is similar to how our brains work; we interpret each word in a sentence one after the other, using context from earlier words to understand the meaning as we go. RNNs mimic this process by keeping track of the sequence they’re processing, which allows them to excel in tasks like natural language processing.
For example, Google uses RNNs to rank search results by understanding the sequence and context of what a user might be looking for. Instead of showing you random results, it organizes them based on relevance, using prior search data to help predict what’s useful to you. RNNs’ ability to retain context makes them a natural choice for such tasks where the order of information matters.
2. Memory & Feedback Loops – Leveraging Past Data for Better Predictions
RNNs have what’s called a “feedback loop,” which lets them keep information in a kind of memory as they process new data. This enables them to use past knowledge to make better decisions about the future. Picture it like having a mental note of past experiences that informs your next decision.
In real-world applications, this memory feature makes RNNs perfect for recommendation systems. Netflix, for instance, leverages RNNs to predict what shows you might like based on your previous viewing history. This predictive ability allows Netflix to refine its recommendations continuously, improving its accuracy by around 25%. For users, this results in a more personalized experience, where the platform learns from your preferences over time.
3. Dynamic Problem Solving – Adapting in Real-Time
AI that can learn in real time is key for applications where decisions need to be made on the fly. RNNs are great for this because they adapt as they go, using continuous learning to update their predictions based on new information. This kind of flexibility is particularly useful in dynamic environments where conditions can change quickly.
A perfect example of this is Tesla’s Autopilot. Tesla vehicles use RNNs to help interpret driving conditions, find roads, and respond to changes in real-time like other cars suddenly braking or changing lanes. RNNs help the car’s AI learn from each new experience, enhancing its ability to make accurate, fast decisions while driving. This adaptability is crucial in a field like autonomous driving, where unexpected changes require instant, reliable responses.
4. Temporal Mastery – Understanding Time-Based Patterns and Trends
RNNs have a unique ability to identify patterns that occur over time, which is especially important in areas like forecasting and behavior analysis. They don’t just look at static data but also analyze how things change from one point to the next, capturing what’s called “temporal dependencies.” For instance, this ability is incredibly helpful in applications where predictions depend on historical data.
Amazon uses RNNs to predict demand for products, factoring in time-based patterns like seasonality or even daily fluctuations. By understanding these temporal dependencies, Amazon can forecast with 30% higher accuracy, helping them keep popular items in stock while minimizing overstock. This application of RNNs exemplifies how one-way time-based learning makes these models essential in business and retail.
Why RNNs Are Key for AGI
As we strive to build AGI—AI that can think and reason like a human—RNNs bring us a step closer by equipping machines with an ability to learn, remember, and adapt in ways similar to us. For AGI to work, it needs to process experiences sequentially, make decisions based on memory, and continuously improve itself by learning from past interactions. RNNs do all of these things well, making them an extremely important technology for advancing AGI.
Here’s why RNNs are considered game-changers for AGI:
– Memory-Rich Learning: Unlike many other models, RNNs “remember” past information, enabling AGI to form more complex understandings and apply them to new situations.
– Real-Time Adaptation: AGI will need to respond to real-world changes as they happen. RNNs’ ability to update their “thinking” based on real-time data is vital for this.
– Temporal Awareness: Human cognition relies on understanding time and sequence—knowing not just what happened but when it happened. RNNs replicate this by capturing and analyzing time-based dependencies.
The Future with RNNs: Intelligent, Adaptable, and Human-Like AI
The future of AI, especially AGI, relies on creating systems that think and learn like humans, and RNNs are a powerful step toward that vision. By unlocking the ability to understand sequences, remember context, and adapt dynamically, RNNs are helping AI move beyond static tasks and into more complex, nuanced areas where learning happens continuously. The path to AGI is challenging, but RNNs are at the forefront, laying the foundation for AI systems that can grow smarter with every interaction. With their unique abilities, RNNs are more than just a tool—they’re the building blocks of intelligent, human-like AI, enabling us to unlock unprecedented potential in technology and transform how we interact with the digital world. In summary, RNNs are reshaping the future of AI, making it faster, smarter, and more adaptable than ever. As this technology advances, we’re getting closer to a world where AGI isn’t just an idea but a reality—an AI that learns, evolves, and empowers us to solve complex challenges.