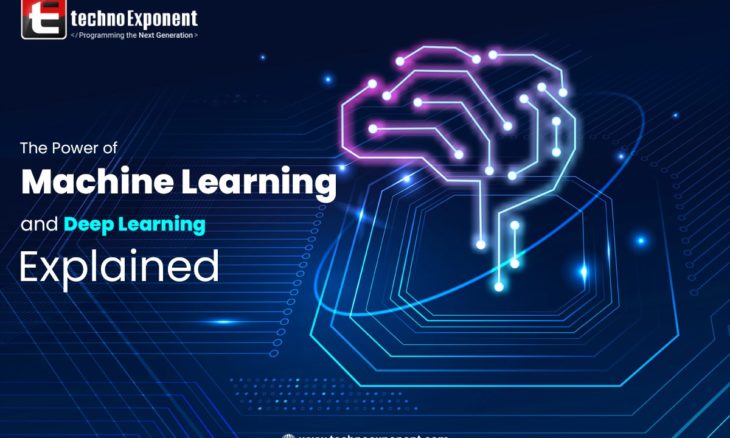
Machine Learning has been an advantageous field for decades, and its importance has significantly grown in the contemporary era of Big Data. The reason for this surge in demand is quite straightforward: organizations require assistance in effectively managing and harnessing the vast and continuous streams of data generated by our systems. Machine learning technology plays a pivotal role by enabling businesses to develop automated models capable of swiftly processing immense datasets and, importantly, acquiring the capacity to “learn” from this data to tackle various challenges. Let us delve into some of the applications of machine learning in the corporate landscape.
Various Usages of Machine Learning in a Nutshell:
Machine Learning finds a multitude of applications across various domains, encompassing our daily lives in numerous ways:
1. Recommendations:
Prominent streaming platforms such as Spotify and Netflix employ Machine Learning algorithms to suggest content based on users’ past preferences and habits, offering personalized viewing and listening experiences.
2. Fraud Detection:
Financial institutions, including banks, utilize Machine Learning models to detect unusual transactions by analyzing parameters like purchase amounts and user locations, promptly alerting customers to potentially fraudulent activities.
3. Search Engine Results:
Leading search engines like Google employ Machine Learning algorithms to tailor search results to individual user behavior. For example, if a user spends substantial time on a lower-ranked webpage, the algorithm may elevate it in subsequent searches related to similar topics.
4. Chatbots:
AI-driven chatbots assist users in resolving issues online by employing trained Machine Learning models to provide automated and contextually relevant responses based on user input.
5. Spam Filters:
Machine Learning algorithms, utilizing neural networks, scrutinize email subject lines, content, and return addresses to safeguard users’ inboxes from unwanted spam emails.
6. Customer Retention:
Service providers leverage Machine Learning models to identify customers displaying signs of potential churn. Strategies such as offering reduced APRs to credit card users who have decreased their card usage are a result of Machine Learning-driven customer retention efforts.
7. Sentiment Analysis:
Also known as opinion mining or emotion AI, sentiment analysis employs natural language processing and Machine Learning to discern the sentiment expressed in social media posts. This analysis aids businesses in understanding public sentiment toward their brand or products.
8. Real Estate Valuation:
Machine Learning algorithms estimate the current value of real estate properties by analyzing features and comparing sales data of similar properties in the vicinity. Platforms like Zillow and Redfin utilize this technology for property valuation.
9. Learning Apps:
Educational platforms like Duolingo harness Machine Learning models to analyze user data and adapt course pacing to individual needs, enhancing the effectiveness of language learning and other educational endeavors.
10. Medical Image Processing:
In healthcare, Machine Learning radiology platforms are trained to identify potential anomalies in patient X-rays, flagging cases that require further medical attention, thus contributing to more accurate diagnoses.
The widespread adoption of Machine Learning technology continues to influence and improve various aspects of our daily lives and industries across the board.
Deep Learning and its Usage:
Deep Learning represents a prominent facet of Artificial Intelligence (AI) that has garnered substantial attention in recent years. This paradigm centers on the notion that computer programs can effectively ingest extensive datasets, analyze them comprehensively, and subsequently provide solutions. This process involves the dissection of complex problems into smaller components, followed by the utilization of neural networks to deduce optimal solutions. Deep Learning finds application in various domains, including but not limited to autonomous vehicles, medical diagnostics, and image recognition. Deep Learning constitutes a subset of Machine Learning, a pivotal field within the domain of AI. The distinction between Deep Learning and Machine Learning lies in the fact that the latter serves as an overarching term encompassing any learning system employing models for data processing, while Deep Learning specifically concentrates on models characterized by multiple levels of abstraction.
Deep Learning has assumed an increasingly pivotal role within enterprises as they seek avenues for innovation and growth. Here are several ways in which Deep Learning can be effectively leveraged within your enterprise:
1. Data-Driven Decision-Making:
Deep Learning empowers organizations to make data-driven decisions, particularly beneficial when dealing with substantial volumes of data that necessitate swift decision-making.
2. Efficiency in Model Building:
Deep Learning streamlines the process of constructing intricate models, requiring only a few lines of code. This obviates the need to develop algorithms from scratch, permitting the use of existing libraries or the creation of custom solutions tailored to specific requirements.
3. Image Recognition:
Deep Learning excels in image recognition tasks, proving invaluable for applications such as facial recognition software or the identification of objects within images and videos.
4. Customer Behavior Insights:
Enterprises can harness Deep Learning to gain profound insights into customer behavior. For instance, predictive analytics can be employed to forecast product popularity, enabling more informed marketing strategies.
5. Task Automation:
Deep Learning facilitates the automation of repetitive tasks, yielding cost savings by reducing reliance on human resources and manual labor.
6. Enhanced Customer Service:
By employing Deep Learning, companies can enhance customer service through improved product or service recommendations, drawing on past interactions and purchase histories to provide more personalized offerings.
7. Data Analysis:
Deep Learning proves invaluable in analyzing data pertaining to various aspects of an enterprise, be it foot traffic in a retail store or customer demographics. This analysis informs strategic decisions and operational optimizations.
8. Custom AI Models:
Deep Learning empowers organizations to develop customized AI models that enable predictive insights into customer behavior, offering a level of precision and depth of analysis previously unattainable.
9. Efficiency Improvements:
By automating tasks that once necessitated human intervention, Deep Learning contributes to efficiency enhancements. Examples include the automated identification of spam emails or the categorization of images into predefined classes, such as “people” or “cars.”
The integration of Deep Learning into enterprise operations presents a multitude of opportunities to enhance decision-making, efficiency, and customer engagement, ultimately fostering growth and innovation.
Techno Exponent offers cutting-edge Machine Learning and Deep Learning services, empowering clients with advanced AI solutions. Their expertise enables businesses to harness the full potential of these technologies for data-driven decision-making, automation, and innovative applications, ensuring superior outcomes in the ever-evolving digital landscape.