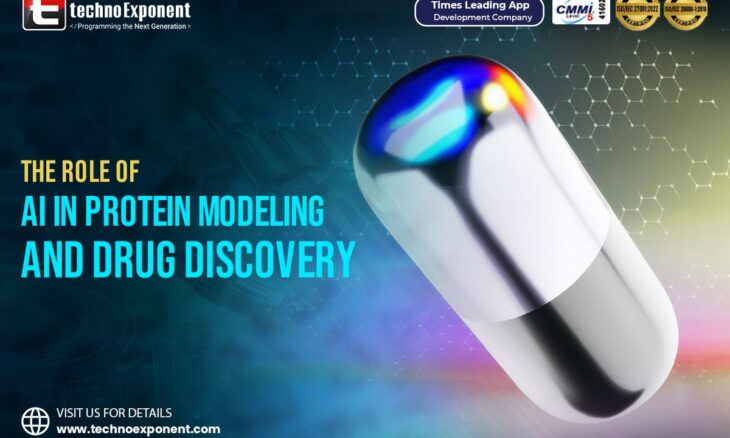
Introduction
Artificial Intelligence (AI) is reshaping scientific research in numerous fields, with protein modeling and drug discovery standing out as two of the most impactful areas. AI-powered techniques are significantly accelerating the process of understanding protein structures and interactions, reducing the time and cost required for drug development. By leveraging machine learning algorithms and computational biology, AI is transforming how scientists identify therapeutic targets, optimize drug candidates, and design novel proteins for medical and industrial applications.
AI in Protein Modeling
Proteins are essential molecules that drive biological processes. Understanding their structure and function is critical for designing new therapies, industrial enzymes, and synthetic biological systems. However, protein modeling has historically been an arduous task due to the complex folding patterns and vast combinatorial possibilities of amino acid sequences.
Predicting Protein Structures
One of the most challenging problems in biology has been predicting the three-dimensional structure of proteins from their amino acid sequences. Traditional methods, such as X-ray crystallography and nuclear magnetic resonance (NMR) spectroscopy, are labor-intensive and costly. AI has dramatically changed this process by enabling rapid and accurate predictions.
A major breakthrough came in 2021 with the introduction of AlphaFold, which demonstrated an unprecedented ability to predict protein structures with near-experimental accuracy. AI models now integrate vast datasets of protein sequences and structures, allowing researchers to infer how proteins fold and interact, aiding in the understanding of diseases and the development of targeted therapies.
AI-Powered Protein Engineering
Beyond understanding existing proteins, AI is now being used to design novel proteins with specific properties. AI-driven protein engineering enables researchers to create proteins optimized for therapeutic, industrial, or environmental applications.
By leveraging machine learning models, scientists can predict how amino acid sequences influence a protein’s function. Some approaches, such as Protein Language Models, treat protein sequences as a computational language, identifying patterns and modifications that improve stability, binding affinity, or catalytic efficiency. This is particularly useful in enzyme engineering, where AI can optimize protein structures to enhance their functionality in industrial settings.
AI in Drug Discovery
Traditional drug discovery is a lengthy and costly process, often taking over a decade to bring a new drug to market. AI is streamlining this process by improving target identification, optimizing drug design, and enhancing clinical trials.
Identifying New Drug Candidates
AI algorithms analyze extensive biological datasets, identifying molecules that could serve as potential drug candidates. Unlike conventional high-throughput screening methods that rely on brute-force testing, AI can predict molecular interactions based on known chemical and biological properties. By simulating how compounds interact with specific targets, researchers can rapidly narrow down promising candidates for further study.
AI models can also predict the three-dimensional interactions between proteins and small molecules, reducing the need for physical trial-and-error experiments. This speeds up the discovery of new therapeutics, particularly in fields such as oncology, neurodegenerative diseases, and rare genetic disorders.
Predicting Drug-Target Interactions
One of the most critical challenges in drug discovery is ensuring that a drug interacts effectively with its intended target while minimizing side effects. AI-powered predictive models analyze vast repositories of known drug-target interactions, enabling researchers to infer how new compounds will behave.
These models also aid in predicting pharmacokinetics and pharmacodynamics, providing insights into how a drug is absorbed, distributed, metabolized, and excreted (ADME). By improving early-stage predictions, AI helps reduce the likelihood of failures in later clinical trials.
Optimizing Drug Design
AI is playing a crucial role in optimizing the molecular structures of drug candidates. Machine learning models can evaluate chemical modifications that improve a drug’s efficacy, stability, and safety. By simulating molecular interactions, AI enables researchers to refine drug structures before synthesizing them in the lab.
This approach is particularly effective in antibody-based therapies and personalized medicine, where drugs must be precisely tuned to target specific biomolecules. AI also aids in de novo drug design, generating novel molecular structures that fit a desired pharmacological profile.
Enhancing Clinical Trials
AI-driven analytics are transforming clinical trials by optimizing patient selection, predicting trial outcomes, and identifying potential safety concerns in real-time. By analyzing electronic health records, genomics, and real-world data, AI can match patients with trials more effectively, ensuring that the right candidates are enrolled for a given treatment.
Additionally, AI systems can monitor patient responses during trials, flagging adverse effects early and allowing for data-driven adjustments. This increases the efficiency of clinical trials, reducing both costs and the time required to bring new treatments to market.
Drug Repurposing
Finding new uses for existing drugs is another area where AI is proving invaluable. By analyzing datasets on drug interactions and disease mechanisms, AI can predict whether approved drugs might be effective against different conditions. This approach has been instrumental in identifying treatments for emerging diseases, including efforts during the COVID-19 pandemic.
Applications Across Industries
While AI-driven protein modeling and drug discovery have clear implications for the pharmaceutical industry, their impact extends beyond medicine. Industrial and environmental applications are also benefitting from AI-designed proteins and enzymes.
Oncology
AI is improving cancer treatment by identifying biomarkers that predict patient responses to therapies. Personalized medicine approaches use AI to tailor treatments based on a patient’s unique genetic profile, improving outcomes while reducing side effects.
Rare Diseases
For conditions with limited treatment options, AI accelerates the identification of potential therapeutic targets. By analyzing genetic and clinical data, AI helps researchers develop treatments for diseases that might otherwise be overlooked due to their rarity.
Infectious Diseases
AI played a critical role in addressing the COVID-19 pandemic by helping researchers analyze viral genome sequences, predict mutations, and design antiviral compounds. AI-based models continue to aid in the rapid response to emerging infectious threats.
Industrial Biotechnology
Beyond healthcare, AI-driven protein design is revolutionizing industrial applications. Companies are using AI to create enzymes that improve the efficiency of chemical manufacturing, food processing, and environmental sustainability. These bio-catalysts enable the production of high-purity compounds and enhance manufacturing processes across multiple sectors.
Challenges and Ethical Considerations
Despite its potential, AI-based protein modeling and drug discovery face several challenges. The quality and accuracy of training data are crucial, as biased or incomplete datasets can lead to misleading predictions. Researchers must ensure that AI models are trained on diverse, high-quality datasets to maximize reliability.
Ethical considerations also play a role, particularly regarding patient data privacy, security, and transparency. Regulatory agencies are beginning to establish guidelines for AI-driven drug development, ensuring that these technologies are used responsibly.
The Future of AI in Protein Modeling and Drug Discovery
Advancements in AI will continue to refine protein modeling and drug discovery. Innovations such as explainable AI (XAI) will enhance transparency in AI-driven decision-making, addressing concerns about interpretability. Additionally, the integration of AI with quantum computing and synthetic biology promises to push the boundaries of what is possible in biopharmaceutical research.
As AI tools become more sophisticated, their role in scientific discovery will expand, opening new frontiers in medicine, industrial biotechnology, and environmental sustainability. Researchers and industry leaders must work together to harness AI’s full potential while addressing its challenges, ensuring that these technological advances benefit society as a whole.
Conclusion
AI is reshaping the fields of protein modeling and drug discovery, accelerating research, improving precision, and reducing costs. By leveraging machine learning algorithms and computational techniques, scientists are uncovering new ways to design proteins, identify drug candidates, and optimize therapeutics. As the field progresses, AI will continue to unlock new possibilities, offering powerful solutions for some of humanity’s most pressing challenges.